Regression analysis is a potent statistical technique used in many fields, including business, economics, psychology, and sociology, to comprehend the relationship between variables. However, learning regression analysis can be extremely difficult for students. Regression analysis homework can be challenging, and students frequently run into a number of roadblocks that prevent them from moving forwards. In this blog from SPSS Homework Help, we will examine the 18 typical challenges that students face when working on challenging regression analysis homework and provide helpful advice on how to get past them. Students can improve their comprehension and competence in regression analysis by confronting these difficulties head-on. Students will leave our thorough investigation with the skills and knowledge needed to successfully negotiate the challenges of regression analysis tasks. This blog aims to offer helpful insights and solutions to empower students in their regression analysis journey, whether it involves understanding problem statements, choosing suitable regression models, preprocessing data, or interpreting regression coefficients. Students can complete their regression analysis homework with confidence and advance their analytical skills if they have a thorough understanding of these typical roadblocks and the advice provided.
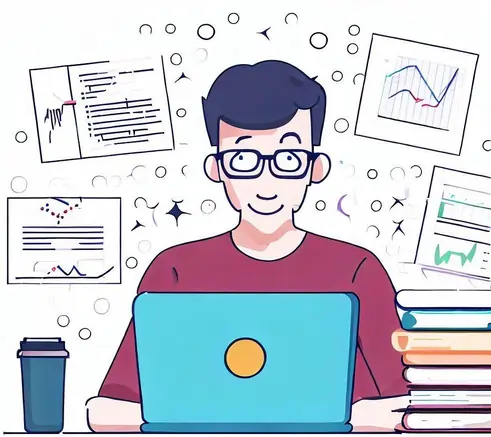
- Understanding the Problem Statement
- Selecting the Appropriate Regression Model
- Data Collection and Preprocessing
- Identifying and Addressing Multicollinearity
- Checking for Linearity and Transforming Variables
- Handling Heteroscedasticity
- Assessing Model Fit and Goodness of Fit Measures
- Interpreting Regression Coefficients
- Dealing with Autocorrelation
- Addressing Endogeneity
- Explaining Statistical Assumptions
- Developing Hypotheses and Testing Significance
- Managing Time and Deadlines
- Seeking Help and Clarification
- Practicing with Real-Life Examples
- Reviewing and Revising Solutions
- Enhancing Data Visualization Skills
- Developing a Growth Mindset
Understanding the problem statement is the first challenge that students encounter. Complex problems involving assumptions and multiple variables can arise during regression analysis. It may be difficult for students to understand the fundamental idea and determine what the question is asking for. To overcome this difficulty, carefully read the problem statement, list the relevant variables, and divide the issue into more manageable chunks. You should pay close attention to the requirements and key terms listed in the problem statement because they offer helpful hints for resolving the regression analysis issue. By segmenting the issue into smaller parts, students can approach each one methodically and guarantee a thorough comprehension of the challenge at hand. Additionally, you can get more information and help better understand the problem statement by consulting textbooks, and online sources, or asking your instructors for advice. To move forwards with the regression analysis and create a workable action plan, a clear understanding of the issue must be developed.
For a thorough analysis, selecting the appropriate regression model is crucial. Due to the availability of numerous options, including logistic regression, multiple regression, and linear regression, students frequently struggle to choose the best model. Making an informed choice requires a thorough understanding of the problem's requirements as well as the nature of the data. Assess the relationships between the dependent and independent variables first. Take into account the kind of data being analyzed, such as continuous, categorical, or binary results. While logistic regression is appropriate for binary outcomes, linear regression is frequently used for continuous outcomes. When several independent variables have an impact on the dependent variable, multiple regression is used. Consider each regression model's underlying assumptions as well, and determine whether the assumptions are supported by the available data. Students should carefully consider and comprehend the context of the problem before choosing the best regression model for their analysis.
Regression analysis requires the collection and preprocessing of data. The collection of pertinent and trustworthy data, data cleaning, and handling of missing values or outliers may present difficulties for students. Make sure you have access to a diverse and representative dataset, use effective data cleaning techniques, and handle missing values and outliers correctly to combat this. Start by determining the data-collection sources, such as surveys, databases, or experiments. Make sure the information gathered is pertinent to the research question and contains all required variables. After that, thoroughly clean the data by eliminating any duplicates, resolving any discrepancies, and handling any missing values. For example, imputation or excluding cases with missing values based on the degree of missingness can be used to handle missing values. Outliers, which are extreme or unusual data points, should also be addressed by being recognized, understood, and evaluated for their impact on the analysis before being retained or eliminated. For the results of regression analysis to be accurate and reliable, proper data collection and preprocessing are essential.
When independent variables in a regression model have a high degree of correlation, multicollinearity occurs, producing results that are unstable and unreliable. Identification and correction of multicollinearity are frequently difficult for students. Perform a correlation analysis between the variables, and take into account methods like the variance inflation factor (VIF) to identify and manage multicollinearity. To find pairs that are highly correlated, begin by calculating the correlation coefficients between independent variables. Strong linear relationships are indicated by correlation coefficients that are close to 1 or -1. Regression coefficient interpretation can be impacted by high multicollinearity, which can produce unreliable results. Consider methods like VIF, which evaluates the degree of correlation between each independent variable and other variables in the model, to address multicollinearity. One of the correlated variables may be removed, or variables may be transformed, as potential solutions if VIF values are higher than a predetermined threshold, indicating high multicollinearity. Additional insights into identifying and effectively dealing with multicollinearity can be gained by consulting statistical textbooks or asking professors for advice.
Regression analysis relies heavily on assumptions like the linearity of relationships between variables. Determining whether the relationship between variables is linear or whether transformations are required may be difficult for students to grasp. To evaluate linearity and transform variables as necessary, use scatter plots, residual plots, or statistical tests. Making scatter plots of the dependent variable versus each independent variable can show whether the relationship is linear visually. The residuals are plotted against the predicted values to create residual plots, which can be used to find any systematic patterns or linearity deviations. The Jarque-Bera test and the Durbin-Watson test are two statistical analyses that can shed light on the linearity assumption. Consider applying transformations to the variables, such as polynomial or logarithmic transformations, to bring about linearity and increase the regression model's accuracy if the linearity assumption is broken.
When error variability varies across all levels of the independent variable, heteroscedasticity is present. For students, dealing with heteroscedasticity can be confusing. Use diagnostic tests to identify heteroscedasticity, such as the Breusch-Pagan or White tests, and take appropriate corrective action into account, such as transforming variables or employing robust regression techniques. Heteroscedasticity can be visually determined by plotting the residuals against the expected values or the independent variables. Statistical proof of heteroscedasticity can be presented by diagnostic tests like the White or Breusch-Pagan tests. Transforming variables, such as by applying the natural logarithm or square root, can help stabilize the variability if heteroscedasticity is present. As an alternative, to take into account heteroscedasticity and produce more accurate estimates, robust regression techniques can be used, such as weighted least squares or robust standard errors.
Regression analysis requires measuring the goodness of fit and assessing model fit. R-squared, adjusted R-squared, and F-statistics are examples of measures that students frequently find difficult to comprehend and interpret. To evaluate the model's quality, become familiar with these measures and how to interpret them in the context of the issue. R-squared measures the percentage of the dependent variable's variance that can be accounted for by the independent variables, while adjusted R-squared takes sample size and the number of variables into account. F-statistics evaluate the regression model's overall significance. It is important to take the problem context, significance level, and domain-specific knowledge into account when interpreting these measures. Keep in mind that a high R-squared or F-statistic should be accompanied by theoretical relevance and robustness; it does not automatically indicate a good model fit.
Another difficulty students encounter is interpreting regression coefficients. Drawing meaningful conclusions requires an understanding of the importance and trend of each coefficient. To interpret the effect of the coefficient on the dependent variable, take note of the p-value, confidence interval, and sign of the coefficient. A significant impact is indicated by a p-value that is less than the selected significance level (for example, 0.05), which indicates the statistical significance of the coefficient. A selection of logical values for the coefficient is provided by the confidence interval. In order to ascertain whether the relationship between the independent and dependent variables is positive or negative, also take into account the sign of the coefficient (+/-). The problem context and theoretical expectations should serve as the foundation for interpretation. When interpreting the findings, it's crucial to avoid assuming causal relationships solely based on regression coefficients and to take other pertinent variables and potential confounders into account.
When errors in a regression model are correlated with one another, autocorrelation happens. Identifying and dealing with autocorrelation may be difficult for students. To detect autocorrelation, use methods like the Durbin-Watson test or plot residuals, and then take corrective measures like including lag variables or using autoregressive models into consideration. By analyzing the correlation between residuals at various lagged time points, the Durbin-Watson test determines whether autocorrelation is present. While values noticeably higher or lower than 2 signify either positive or negative autocorrelation, respectively, values significantly higher or lower than 2 signify no autocorrelation. The residuals can also be plotted against time to reveal patterns that could be autocorrelation. Include lagged variables as additional independent variables in the model to address autocorrelation, or think about using autoregressive models like ARIMA or ARCH models, which are designed to handle autocorrelated errors.
When the independent variables and the error term are correlated, endogeneity develops, resulting in inaccurate and inconsistent regression estimates. It might be difficult for students to discuss endogeneity. Use methods to reduce endogeneity and get accurate results, such as instrumental variables, difference-in-differences, or propensity score matching. When there are variables that are correlated with the endogenous variable but not with the error term, instrumental variables can be used as stand-ins to address endogeneity. In order to lessen the impact of unobserved factors, difference-in-differences compare the treatment group to the control group over time. By matching treated and control groups based on their propensity scores, propensity score matching ensures comparability. These methods offer more reliable estimates of the relationships between the independent and dependent variables and help allay endogeneity concerns.
The absence of outliers, the independence of the errors, and the normality of the residuals are some of the assumptions underpinning regression analysis. These presumptions may be challenging for students to comprehend and defend. To satisfy the statistical assumptions, carefully consider each premise, apply diagnostic tests, and offer suitable justifications. Diagnostic tests that can be used to evaluate the validity of these presumptions include the Durbin-Watson test for independence and the Shapiro-Wilk test for normality. Based on theoretical predictions and the characteristics of the data, defend the assumptions. For instance, while the independence assumption ensures objective coefficient estimates, the normality assumption ensures the validity of statistical tests and confidence intervals. To ensure accurate results, outliers, significant observations, and assumption violations should be handled properly. For a regression analysis to be accurate and valid, it is essential to have a thorough understanding of these assumptions and their implications.
Regression analysis includes the creation of hypotheses and the evaluation of their significance. The formulation of sound hypotheses and the execution of hypothesis tests may prove challenging for students. Use appropriate statistical tests, such as t-tests or ANOVA, to determine significance. Clearly state the null and alternative hypotheses in light of the problem context. The alternative hypothesis asserts the existence of a relationship or difference, whereas the null hypothesis asserts their absence. Depending on the nature of the research question and the types of variables involved, choose the appropriate statistical test. T-tests, for instance, can be used to compare the means of two groups, whereas ANOVA tests can evaluate differences between several groups. By comparing the p-value to the predetermined significance level, analyze the test results. A p-value that is less than the significance level denotes statistical significance and offers proof either in favor of or against the hypotheses.
When working on challenging homework involving regression analysis, time management is essential. Students frequently struggle to set aside enough time and meet deadlines. To ensure timely completion, make a realistic study schedule, divide the workload into manageable chunks, and prioritize wisely. Determine how much time will be needed for each task, and schedule specific study sessions accordingly. Take into account elements like the homework complexity, your familiarity with the subject, and any other commitments. By dividing the tasks into smaller, more manageable chunks, it is possible to avoid feeling overwhelmed and to be more productive. Set due dates for every task as well to monitor your progress and make sure you stay on course. Productivity and efficiency can also be increased by using time management techniques like the Pomodoro Technique, which involves working in focused intervals and taking brief breaks.
When faced with challenges, students might be reluctant to ask for assistance or clarification. Overcoming this obstacle and actively seeking help from professors, peers, or online resources is crucial. Understanding and problem-solving skills can be significantly improved through collaborative learning and doubt clarification. Speak with your professors or teaching assistants if you need assistance or clarification on certain ideas or issues. Join classmates in group study sessions or discussions to share ideas and discuss problems. Additional explanations and examples can be found on educational websites, tutorial videos, and online discussion forums. Always remember that asking for assistance is not a sign of weakness but rather an active strategy to accelerate your learning and successfully navigate challenges.
Regression analysis concepts can be better understood by using real-world examples. Students occasionally struggle to apply their theoretical knowledge in real-world situations. Look for pertinent case studies, industry examples, or research papers to gain knowledge about regression analysis's practical applications. Regression analysis is used in many different scenarios and fields, which can help you better understand the concept and make it more relatable. Examine how regression models are used to forecast business outcomes, analyze economic trends, and forecast consumer behavior. To find case studies and real-world examples, look through academic journals, business publications, or data science blogs. To better understand the concepts and hone your problem-solving abilities, practice resolving regression analysis issues based on these examples.
For regression analysis homework to be of higher quality, reviewing and revising solutions is essential. Due to time constraints or a lack of thoroughness, students frequently overlook this step. Give yourself enough time to review your answers, check your math thoroughly, confirm your interpretations, and make any necessary corrections. Pay close attention to your calculations, making sure that the data entry, variable coding, and regression model application are accurate. Check the statistical results to see if they match the requirements of the problem. Examine your interpretations critically, making sure that they follow the logic and are backed up by data from the analysis. To learn more and find areas for improvement, think about asking your peers or teachers for feedback. Utilize this criticism to improve the clarity and coherence of your solutions. Regression analysis homework solutions that are more accurate and comprehensive are produced when you take the time to review and revise your work.
Understanding and presenting the findings of a regression analysis can be facilitated by effective data visualization. Students might find it difficult to make charts and graphs that are instructive. To improve your data presentation abilities and make your analysis more aesthetically pleasing, practice using visualization tools like Matplotlib, ggplot, or Excel. Investigate various plot types, such as scatter plots, line plots, bar charts, or heatmaps, to illustrate the relationships between variables and draw attention to important findings. For information to be communicated clearly and accurately, pay attention to labeling, color selections, and appropriate scaling. By highlighting trends, patterns, or outliers in the data, visualizations can be used to supplement regression analysis. To improve your capacity to effectively communicate the results, practice interpreting and explaining the visuals.
The biggest difficulty students encounter is keeping a growth mindset while they are learning. It can be difficult to perform regression analysis, and failures are inevitable. Accept setbacks as opportunities for learning, take responsibility for your errors, and keep working to advance your regression analysis abilities. Recognize that regression analysis requires practice, repetition, and ongoing learning to master. Consider challenges as a means of improvement rather than as a source of discouragement. Set reasonable goals to track your progress, reflect on your actions, and ask professors or peers for their opinions. Instead of concentrating solely on the results, place more emphasis on the learning process. Accept the importance of patience, resiliency, and flexibility when working on challenging regression analysis homework. You can overcome challenges, increase your knowledge, and master regression analysis with a growth mindset, laying a strong foundation for your academic and professional success.
Conclusion
In conclusion, completing difficult regression analysis homework successfully necessitates a combination of tenacity, critical thinking, and a solid understanding of statistical concepts. Students' ability to create accurate and insightful regression analysis solutions can be greatly improved by recognizing and proactively addressing the common challenges described in this blog. It is essential to keep in mind that mastering regression analysis requires consistent practice and the promotion of a growth mindset. Success in a variety of academic disciplines and research endeavors will be facilitated by embracing challenges as chances for growth, learning from mistakes, and maintaining a positive attitude. Students can improve their regression analysis abilities with hard work and by using the advice in this blog, gain confidence in their capacity to handle challenging situations. In the end, learning regression analysis holds enormous potential for both professional and personal advancement, opening doors to fresh possibilities and broadening horizons in the field of data analysis and interpretation.